Want to Really Understand AI? Take a Look Back into History
Artificial Intelligence (AI) might seem like a recent innovation, given its rise in headlines and discussions. However, AI has been evolving for over 70 years. To fully appreciate the technology shaping industries today, it helps to explore how AI began, the key breakthroughs along the way, and where it might lead us next.
The Early Days: Pioneers and Concepts
The story of AI starts with a fundamental question posed by Alan Turing (Yes, from the movie with Benedict Cumberbatch) in 1950: “Can machines think?” His proposal of the "imitation game," now known as the Turing Test, set the foundation for evaluating machine intelligence. This was followed five years later by the coining of the term "artificial intelligence" during a Dartmouth research proposal.
The initial focus of AI was symbolic reasoning, leading to the development of expert systems in the 1960s. These systems captured human expertise in specific fields and applied it to solve problems like diagnosing medical conditions or identifying organic molecules. By the 1980s, these systems were saving companies millions and were a popular tool across industries.
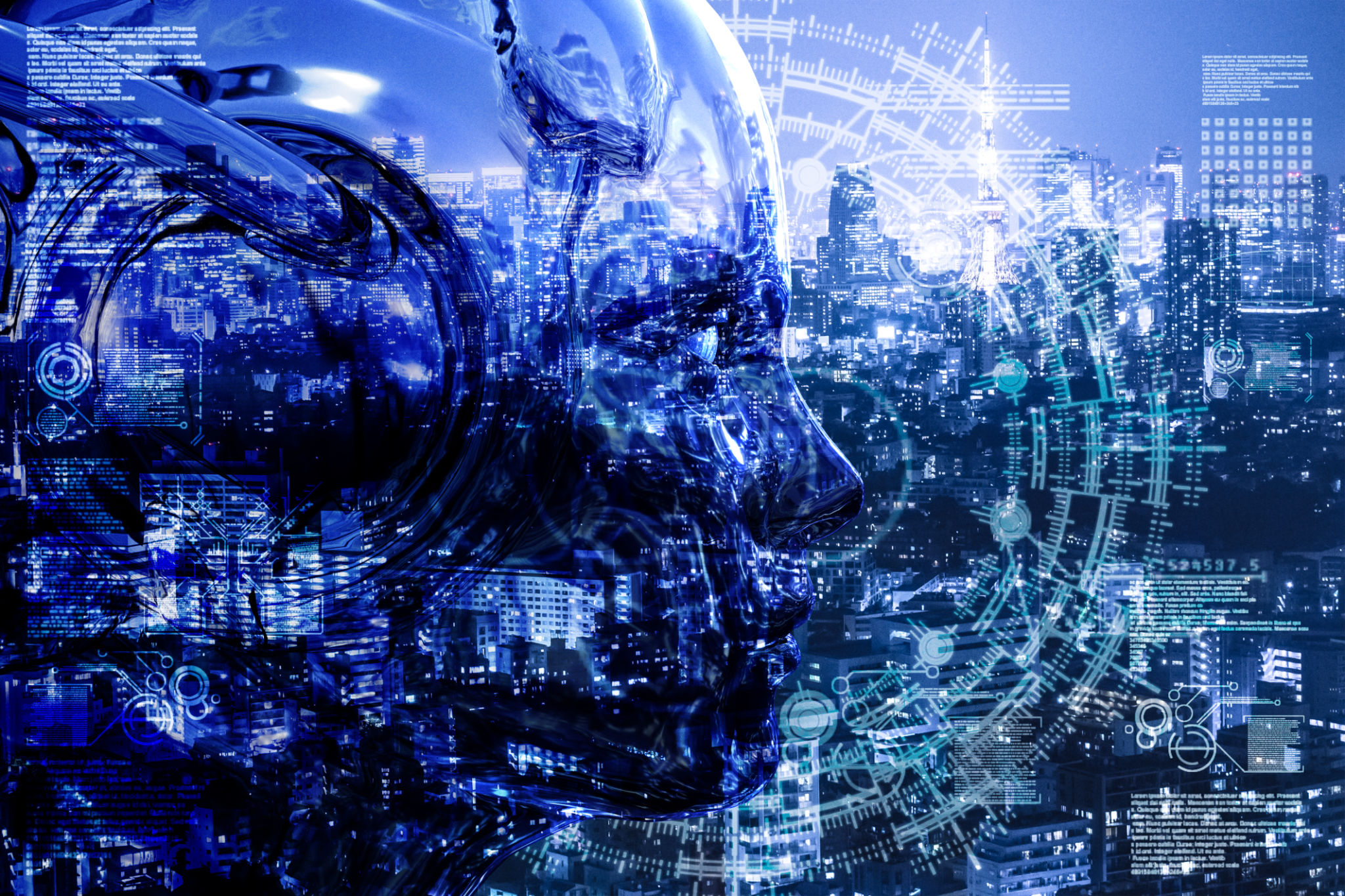
The Neural Network Breakthrough
In parallel, another field of AI was taking shape: connectionism. Instead of mimicking human reasoning, connectionism aimed to replicate the structure of the human brain. Early mathematical models of neurons paved the way, but practical advancements didn’t emerge until the 1980s with the creation of the multi-layered perceptron (MLP).
This model allowed machines to learn from data, adapting and improving through numerical weightings in its connections. The MLP’s ability to classify new, unseen data made it a game-changer, particularly for applications like handwritten character recognition.

Modern Advancements: Generative Models and Transformers
Building on the success of earlier neural networks, newer models began to tackle more complex tasks. The convolutional neural network (CNN), developed in 1998, removed the need for pre-processed inputs by automatically identifying key features of images.
In recent years, generative neural networks like GANs (Generative Adversarial Networks) and transformer networks have transformed what AI can achieve. GANs produce high-quality content by incorporating a built-in critic, while transformer networks, exemplified by tools like GPT-4, use vast datasets to generate text, images, and even music.
Unlike earlier systems focused on narrow domains, these newer models are generalised, capable of tackling almost any topic and assisting across industries.
What AI Can (and Cannot) Do
Generative AI’s rapid development has prompted concerns about its impact. Predictions of AI surpassing human intelligence are common in popular media but remain far from reality. AI systems are improving in capability, reliability, and accuracy—not in achieving consciousness. As one expert remarked, the Hollywood fantasy of conscious machines remains a distant dream.
AI excels in tasks like pattern recognition, data classification, and content generation. Yet symbolic AI, which incorporates structured knowledge, continues to play a vital role. For instance, driverless cars benefit from programmed rules of the road, while medical AI systems combine learned patterns with verified medical knowledge for reliable diagnoses.
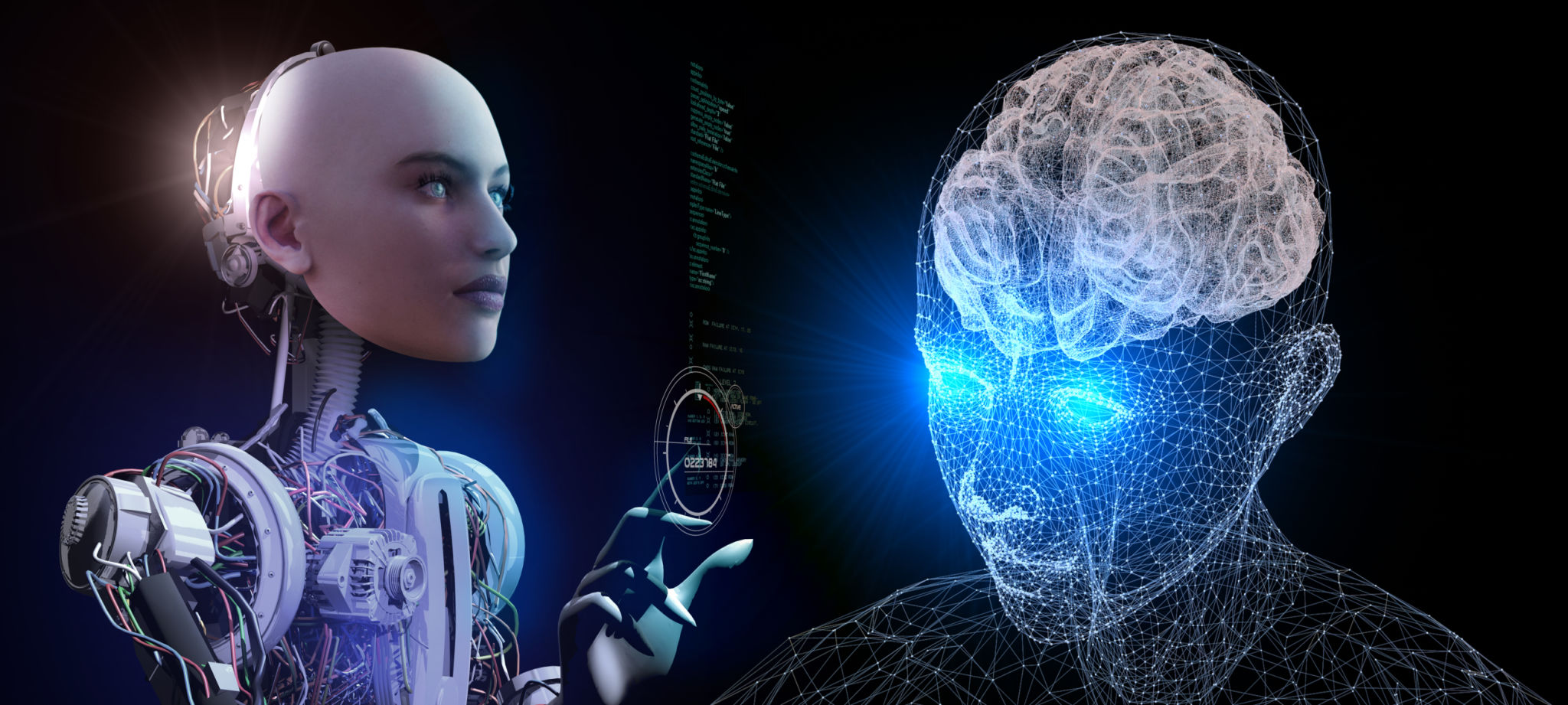
Where AI Is Heading
The future of AI looks promising. And frightening if you are no prepared personally or professionally...more on this later...
Its applications span industries, from healthcare to logistics, improving efficiency and enabling innovation. However, its potential lies not in replacing human roles but in enhancing them. AI systems can automate repetitive tasks, provide insights from large datasets, and even support creative endeavours.
Rather than focusing solely on machine learning, the future will likely involve a combination of techniques. Symbolic AI will continue to support explainability, societal knowledge will help address biases, and advanced neural networks will tackle complex tasks.
A Future of Opportunities
The history of AI shows how far we’ve come—from theoretical questions to practical tools revolutionising industries. Its evolution is a reminder that while AI is powerful, it remains a tool designed to complement human capabilities, not replace them. By understanding its past, we can better navigate its present and prepare for a future where AI continues to open new possibilities.
If you're curious to learn more about the principles behind AI and its potential applications, consider joining our AI Fundamentals Masterclass or AI Bootcamp for a comprehensive introduction. Become part of the AI class of 2025.